AI, Cancer, and HIV
An interview with Dr. Olivier Elemento
Olivier Elemento, PhD, is Director of the Englander Institute for Precision Medicine and Associate Director of the Institute for Computational Biomedicine at Weill Cornell Medicine. His research group combines big data and artificial intelligence with experimentation and genomic profiling to accelerate the discovery of cancer cures. amfAR VP and Director of Research Andrea Gramatica, PhD, spoke with Dr. Elemento about his work and its potential applications in HIV research.
Dr. Andrea Gramatica: Can you tell us about your current research focus and priorities?
Dr. Olivier Elemento: We use genomics [the study of entire sets of genes] and artificial intelligence to try to make medicine more personalized, analyzing clinical samples to understand detailed mechanisms of disease within individual patients. Our main focus is on cancer patients because tissue is easy to access, and there’s lots of technology we can use now to analyze the tissue. Cancer is also a disease that’s often localized to the tissue that’s affected, so it’s easy to find.
But everything we do has applications beyond cancer, and we think about multiple technologies to help us understand cancer and other diseases. One is genomics, where we can sequence somebody’s DNA, or cancer DNA, really quickly and assess all the mutations. And when we do this, we see that every cancer patient is different. I’m actually interested in pushing towards reclassification of cancer essentially as a rare disease, because we’ve never seen two patients with the same mutations. It’s a reason for us to try to individualize treatment.
We also use technology such as RNA analysis to understand which genes are expressed. We use another layer of analysis where we can examine tissue from patients with cancer and other diseases at single cell resolution, understand different cell populations within tissue, and then try to understand how these different populations interact with each other. We’ve also developed ways to grow cells from cancer patients in a dish, make a lot of avatars of people’s tumors, and then, using robotics, test different drugs on these avatars, and essentially get a lot of additional data points about how to better treat the patient.
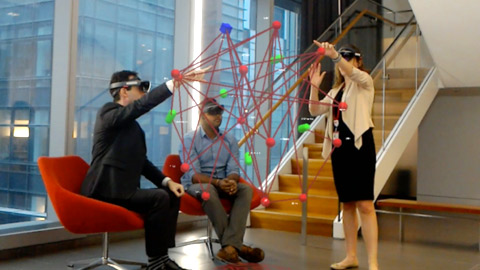
Can you explain what you mean by “avatar” in this context?
It’s not yet something we’ve applied to patients. We’ve applied it in a preclinical setting, but we’re seeing a lot of promise, because we do a lot of predictions and validations, and the validations work really well. So we have a couple of grants out to essentially try to put this into practice in a cohort of patients. Then we can get a sense of whether the combinations that come out of this approach would make sense, and an oncologist would in theory want to use them. Down the line we will have to do an actual trial of effectiveness, but we’re taking it one step at a time.
Are there examples of AI being used effectively in clinical settings?
AI is being used more and more for diagnosis, and the classic case is radiology. A Swedish study that came out a couple of months ago showed that an AI analysis of mammography images was able to identify small cancers of the breast more effectively than a radiologist. It was very clear in this large multicenter trial that AI helping the radiologist was better than a radiologist alone.
Many countries struggle to provide access to the latest healthcare technologies. Are you concerned that the increased use of AI will only exacerbate these inequities?
It’s a major concern. In general, these tools require extensive knowledge about how they work and how to assess the results for reliability, and they also rely on having good data. All of that creates obstacles that can be resolved with investments and resources, but obviously those resources don’t exist in many settings.
You can make the argument that because AI is, in theory, available from anywhere, it can capture some knowledge that can be made available to a broad audience. But the resources needed to make the benefits of AI available are substantial because you need data that goes into the models and you also need skilled users, and internet infrastructure that’s not always available. That’s in addition to the traditional kinds of limitations, such as the expense of many cancer drugs, especially combinations of drugs.
I think down the line, AI is going to make it a lot easier to create new medicines, with the right regulations in place and the right support for innovation. In theory, we can create many more drugs and much more quickly than what we can do now.
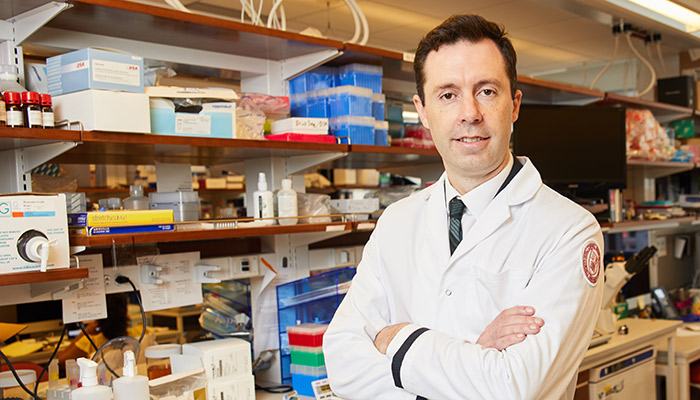
Do you see ways in which this type of work could be applied to treating or curing HIV?
I certainly think so. I think of the work we do in terms of data generation and using AI is essentially, as I say, creating a virtual twin of a patient. And the idea is that once we have a reliable model, you can essentially simulate what would happen to cells if you were to treat them with, say one drug, two drugs, or combinations of drugs. Again, the idea is to observe the cells to see what pathway changes if you treat them with a drug. For example, building a virtual model of HIV-infected T cells could help understand what it would take to activate certain pathways that would make cells susceptible to another drug.
Conceptually, I think a lot of what we do has the potential to apply to HIV as well as other diseases. With cancer, we’re trying to find what cell surface markers they express so that we can design antibodies or CAR T cells to kill those cells specifically. This kind of analysis could also be applicable to HIV to find T cells that are infected and determine what kind of markers they express and how can you help the immune system recognize them.
Where do you see AI-generated advances in medicine heading in the next five or 10 years?
One possibility that’s really exciting to me is rethinking how we develop drugs for individual patients, as opposed to populations, because I really think that’s the future of medicine. We may have some of the tools already that will allow us to do this. I’m pretty sure that soon we’ll be able to have a machine where you input the description of a compound, and then it creates that compound—a machine for 3D printing of small molecules. And then I think we can also more and more rely on the ability to predict what would happen in the body when patients are treated with certain molecules.
Our group has actually done work showing that we can predict results of phase one trials of molecules in cancer and outside of cancer with remarkable accuracy by just looking at the structure of the compound as well as understanding what it does in cells. And we can predict failure or success in phase one trials with a high level of accuracy. In fact, the FDA has announced recently that they are actually quite open to considering that kind of data for new drug applications, and I think that’s kind of game changing. Because they’re saying, well, we may actually not need or even want animal data, for example, if the systems are reliable. We could use organoids [miniature 3D tissue cultures that mimic the structure of organs] or computational toxicity modeling. So that’s opening the door for these models to become better and better and be added to this pipeline for creation of immunized medicine. I’m looking forward to a future where we can essentially create a drug for every patient. And I think that future will happen sooner than we think.
I think that the world is going to move away from animal models. It’s not going to happen overnight, and it’s not going to happen for every drug, but I think there’s a path towards relying more and more on computational models and hopefully not having to use animal models at all going forward.
Click Here to read more from the May 2025 issue of amfAR INNOVATIONS.
Share This: